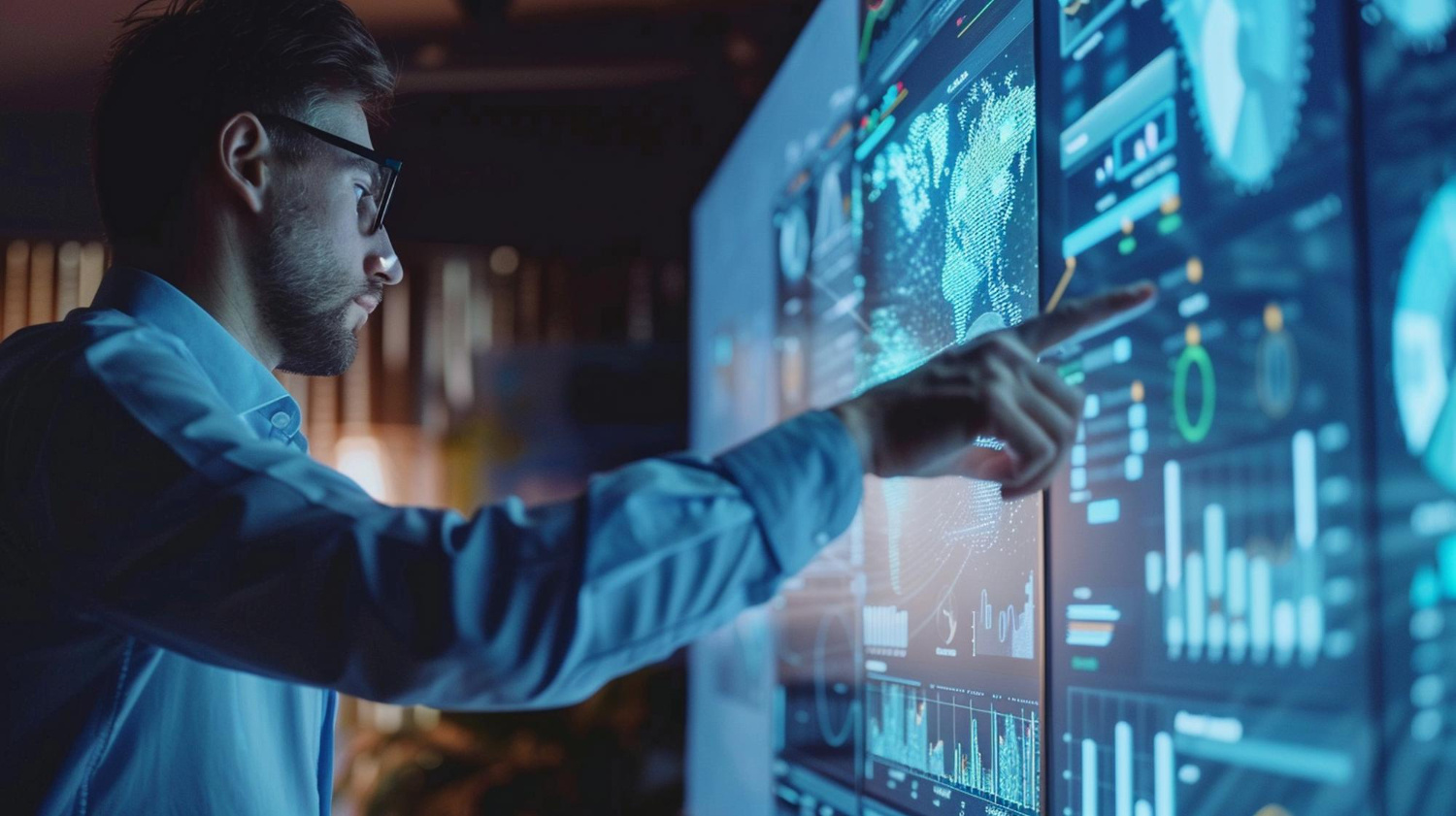
What is Data Processing
The Data Processing Cycle
Types of Data Processing
What Makes Data Processing Effective
In today's scenario, where information is a valuable currency, data processing emerges as the fundamental catalyst for extracting value from the enormous volumes of available data. From business transactions to social media interactions, IoT devices, and scientific data, we are immersed in an ocean of data that requires analysis and interpretation to uncover patterns, identify trends, and make informed decisions. To tackle these challenges, data processing becomes essential, encompassing a wide range of techniques and approaches that allow the manipulation, transformation, and analysis of datasets from various sources and formats.
What is Data Processing?
Data processing consists of a sequence of activities performed logically and orderly, with the aim of transforming scattered data into relevant information for analysis. After all, the mere existence of data is not sufficient; its value arises when it can generate comprehensible and useful patterns.
Although data has always existed and will continue to exist, what truly makes it valuable today is the ability to use it in a qualified manner to understand trends, identify patterns, and develop effective business strategies.
The Data Processing Cycle
Before exploring the different types of data processing, it is crucial to understand each stage of the data processing cycle. This cycle comprises various phases that transform raw data into valuable information.
1. Data Collection
The first stage consists of Data Collection, where data is gathered from reliable sources, including databases, data lakes, and data warehouses. At this point, it is crucial that the data sources are accurate and well-constructed.
2. Data Preparation
In data preparation, also known as preprocessing, raw data is organized and cleaned to facilitate subsequent steps. This phase is essential to ensure that the data is ready for analysis.
In this stage, raw data begins to become readable and provides information. This data is stored in a system, such as a data warehouse, making it ready for analysis.
4. Data Processing
For Data Processing, machine learning and artificial intelligence algorithms are used to interpret the data. The process may vary depending on the source and purpose of the data.
5. Data Output
The second-last stage of the cycle consists of Data Output, where data visualization and presentation become crucial for business analysis. At this point, the data can be used by various sectors of an organization to aid in decision-making.
6. Data Storage
Finally, the Data Storage stage occurs after the analysis, where the information is stored for easy future access and implementation of insights by various members and sectors of the organization.
Types of Data Processing
Given the complexity and diversity of tasks in the data processing cycle, it is essential that professionals have access to a variety of approaches to handle them. Therefore, it is crucial that organizations understand the peculiarities of each method to select and apply the most appropriate type of data processing to their specific needs. The types of data processing include:
- Batch Processing: data is collected and processed in groups, or "batches," at defined intervals. It is temporarily stored until a significant amount is ready for processing. This type of processing is used for tasks that do not require an immediate response, such as payroll processing or generating financial reports.
- Real-Time Processing: unlike batch processing, data is processed as soon as it is received. It is used when an immediate response is non-negotiable, in security monitoring systems or online financial transactions. This type of processing requires a robust infrastructure to handle a large volume of data in real time.
- Online Processing: occurs when there is direct user interaction with a computer system, where data is entered and processed instantly. It is used in web applications, e-commerce systems, and streaming services, where quick response is critical for a satisfactory user experience.
- Offline Processing: involves processing data without a real-time connection to a computer system. This can be done on local systems or remote servers that are not continuously connected to the internet. Offline data processing is used in historical data analysis, predictive modeling, and data preparation for future analyses.
Thus, it is understood that each type of data processing has its own advantages and disadvantages for the business, and the choice of method depends on the needs and characteristics of the data involved.
What Makes Data Processing Effective?
Data processing becomes extremely effective in an organization by transforming large volumes of raw data into actionable information and strategic insights. Using advanced analysis techniques, such as machine learning and artificial intelligence algorithms, companies can identify hidden patterns, market trends, and customer behaviors that would otherwise go unnoticed.
Having detailed knowledge of the data enables more precise and informed decision-making, allowing the organization to respond quickly to market changes, optimize its operations, and develop products and services that better meet customer needs.
NCS Consulting offers specialized data processing methods and services for your organization. Don’t leave your decisions in the dark—contact us and see how we can revolutionize your data management to drive growth and efficiency in your business.
Leave Your Comment Here